Introduction
Did you know that you don't need to be a seasoned data scientist to create wonderous AI models? This has become possible due to the magic of Automated Machine Learning, or simply AutoML.
In the realm of data science and AI, AutoML is steadily becoming a game changer, particularly for those dabbling with Keras and ML projects. Why, you ask?
That's because AutoML handles a plethora of complex tasks like preprocessing, feature engineering, or algorithm selection, thus letting anyone become an AI maestro.
Now you might be wondering if AutoML is just a fancy buzzword or if it truly has substantial benefits.
In this blog post, we're going to walk you through five key benefits of AutoML in data science and AI, offering a greater understanding of why it's causing such a stir. So let's dive straight into the heart of AutoML and find out why it’s making waves in the tech world.
1. Accelerates Model Development
In this section, we'll dive into how technology has transformed the way models are developed, making them faster and more adaptable.
AutoML is at the forefront of this revolution, streamlining many complex processes.
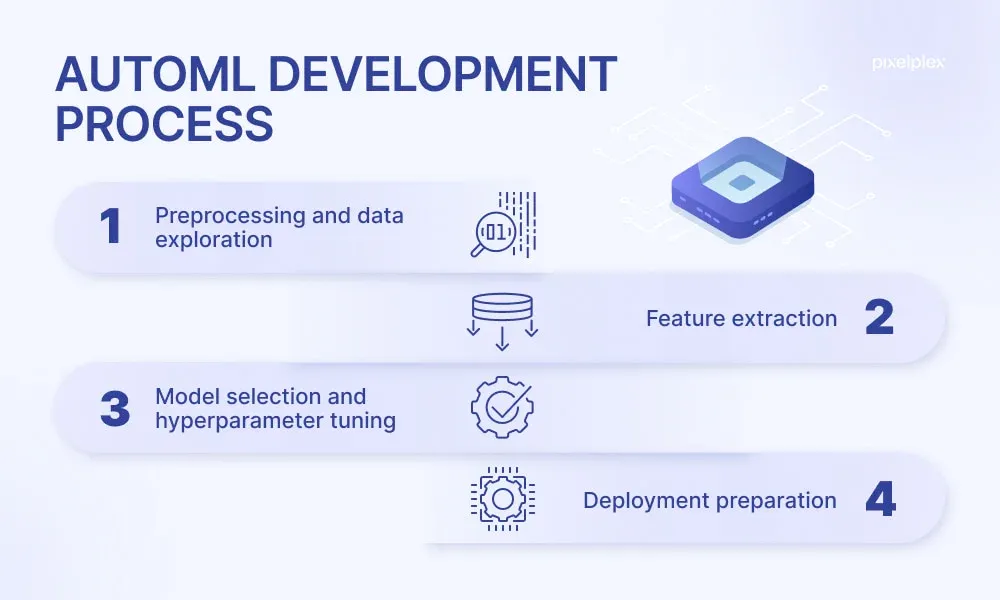
Accelerates Model Development
Traditional model development is akin to meticulously piecing together a thousand-piece puzzle. It requires skill, time, and a lot of patience. Enter AutoML, the game-changer.
AutoML simplifies this process, using algorithms to automatically select the best models and settings, making the development of machine learning models much quicker.
This allows data scientists to spend less time on trial and error, accelerating the overall development process.
Speeding up the Experimentation Phase
Experimentation is critical in developing effective models, but it's traditionally time-consuming.
With AutoML, the experimentation phase is drastically sped up. It automates the tedious aspects of testing various models and tuning parameters, enabling faster iteration and discovery of optimal solutions.
This means reaching valuable insights and achieving project objectives at a significantly quicker pace.
Quick Adaptation to Changing Data Landscapes
The only constant in data science is change. Data landscapes evolve rapidly, and models must adapt quickly to remain accurate and relevant.
AutoML excels in this domain by facilitating quick adjustments to new data patterns. This agility ensures that models remain effective, safeguarding against the risk of obsolescence in fast-moving environments.
Facilitating Rapid Prototyping
Rapid prototyping is crucial for testing ideas and hypotheses in the real world. AutoML serves as a catalyst in this process, allowing for the quick development of prototypes.
This enables teams to validate their approaches and refine their models with real-world feedback, ensuring that the final models are both practical and effective.
Democratizes Data Science
AutoML is breaking down the barriers that once made machine learning the exclusive domain of experts. By automating complex processes, it enables individuals without a deep background in data science to build and deploy effective models.
This democratization is not just about making technology accessible; it's about unleashing the creative potential within organizations, fostering innovation, and bridging the gap between data scientists and domain experts.
2. Democratizes Data Science
Let's explore how AutoML is making data science more accessible to everyone, bridging the gap between complex ML algorithms and non-expert users, and fostering a culture of collaboration and innovation.
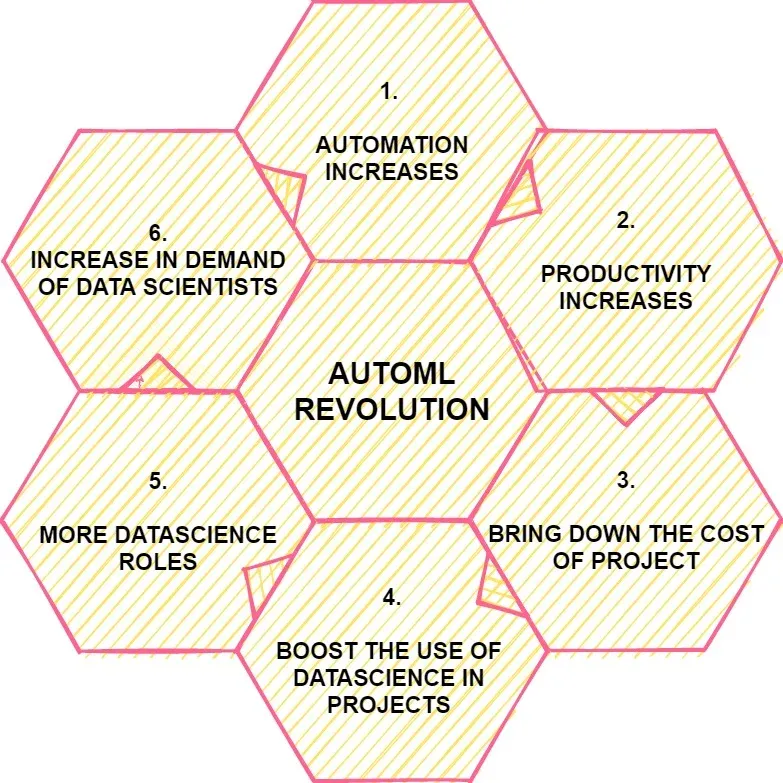
Breaking down complex ML barriers
Machine learning has often seemed like a fortress of complexity, reserved for those with advanced degrees and deep mathematical knowledge.
However, AutoML is changing the game by lowering these barriers, making it possible for a broader range of people to engage in data science.
By doing so, it's not just democratizing knowledge but also empowering more individuals to contribute to the field, irrespective of their academic or professional background.
Empowering Non-Experts to Build and Deploy Models
Imagine being able to construct a sophisticated machine learning model without years of study in computer science or statistics. AutoML is making this possible, transforming non-experts into capable model builders.
This empowerment is revolutionizing industries, allowing professionals to leverage data science for improving decision-making processes, optimizing operations, and much more, all without the need to write complex code.
Enhancing Collaboration between Data Scientists and Domain Experts
Collaboration is key to solving real-world problems, and AutoML is a powerful catalyst. It provides a common ground for data scientists and domain experts to work together seamlessly.
By abstracting the technical complexities, it encourages a focus on solving business problems, enabling a symbiotic relationship where each party brings their strengths to the table - technical prowess from data scientists and deep domain knowledge from the experts.
AutoML Tools Making Data Science Accessible
AutoML tools are the bridge between complex data science concepts and practical application. They provide intuitive interfaces and workflows that guide users through the process of developing machine learning models.
This accessibility is pivotal in breaking down the traditional barriers to entry, allowing a wider audience to engage with, understand, and apply data science in their fields.
Impact on Innovation and Creativity in Organizations
The democratization of data science through AutoML has a profound impact on innovation within organizations.
By enabling individuals across various domains to experiment with data science, it fosters a culture of creativity and experimentation.
This cultural shift can lead to breakthrough innovations as diverse perspectives are applied to solving problems, ultimately driving organizational growth and competitiveness in the digital age.
3. Enhances Model Accuracy and Performance
Now, let's discuss how AutoML not only simplifies the model-building process but also significantly enhances the accuracy and performance of these models through sophisticated optimization techniques.
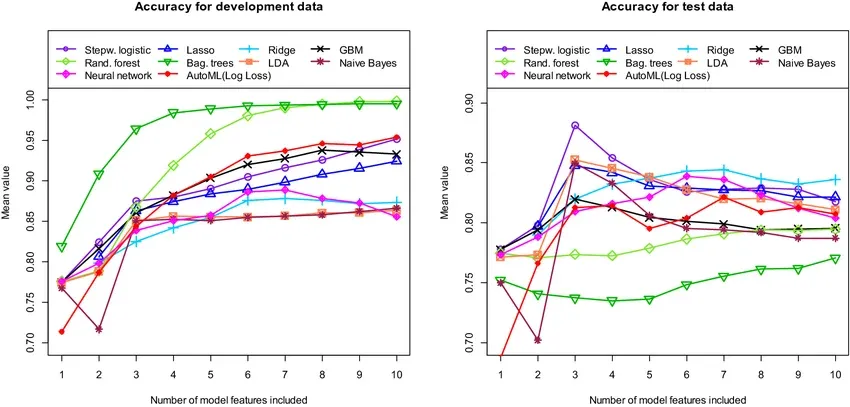
Techniques Used by AutoML for Model Optimization
Optimization is where AutoML truly shines, employing advanced techniques like hyperparameter tuning and algorithm selection to refine models. This process is akin to fine-tuning an instrument to produce the perfect sound.
AutoML’s algorithms meticulously adjust the settings and configurations to ensure the models perform at their peak, often surpassing what a human could achieve through manual adjustments.
AutoML’s Ability to Choose the Right Algorithms and Parameters
Selecting the right algorithm and setting its parameters is more art than science. AutoML automates this process, using data-driven methods to match the problem at hand with the optimal algorithm and parameter configuration.
This capability significantly reduces the guesswork and expertise required, leading to better-performing models that can make more accurate predictions or classifications.
Continuous Learning and Model Improvement
The ability of AutoML to continuously learn and improve models sets it apart. By constantly analyzing performance data and making adjustments, AutoML ensures that models stay relevant and effective over time.
This ongoing improvement process is crucial for adapting to new data trends and maintaining high performance standards.
Benchmarking AutoML Models against Manually Developed Models
Comparison is a critical part of understanding AutoML's value. In side-by-side tests, AutoML models frequently match or exceed the performance of manually developed counterparts.
This benchmarking showcases AutoML's ability to optimize models to such a degree that it can often outpace human experts in developing accurate and efficient solutions.
The Role of AutoML in Competitive Data Science
In the high-stakes world of competitive data science, AutoML has emerged as a key player. It levels the playing field, enabling smaller teams or individuals to compete against well-resourced opponents.
By automating the time-consuming aspects of model development, AutoML allows competitors to focus on strategy and innovation, making competitions more about ingenuity than about who can spend the most time tuning models.
4. Reduces Errors and Bias in Models
Lastly, we'll delve into how AutoML plays a crucial role in minimizing errors and bias, thereby promoting fairness and ensuring models are built on a foundation of quality and integrity.
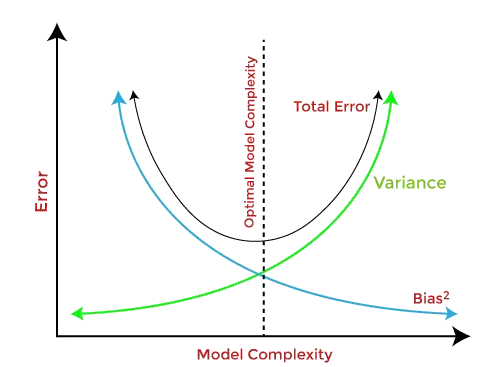
How AutoML Identifies and Mitigates Common Biases
Bias in machine learning models can lead to unfair outcomes. AutoML addresses this by incorporating mechanisms to identify and mitigate common biases.
It evaluates data and model outcomes through a lens of fairness, adjusting processes to ensure that models perform equitably across different groups. This proactive approach is essential for building trust and ensuring the ethical application of AI.
Ensuring Data Quality and Preprocessing Automatically
Data quality is the cornerstone of any model's accuracy. AutoML emphasizes automatic data preprocessing to clean, normalize, and prepare data effectively.
This step is critical in reducing errors and improving model performance, as it ensures that the data fed into the model is of high quality and ready for analysis.
The Importance of Diverse Data Handling Capabilities
Diversity in data is key to capturing the full spectrum of insights and preventing narrow or biased interpretations.
AutoML excels in handling diverse data sets, adapting to various formats and sources without compromising on the quality of insights derived. This capability is crucial for models to be resilient and adaptable to different scenarios.
AutoML’s Contribution to Ethical AI Development
The development of AI must be guided by ethical considerations to ensure its benefits are equitably distributed.
AutoML contributes to this by embedding ethical guidelines into the model development process, from data selection to final deployment. This ensures that models are not just technically sound but also socially responsible.
Addressing Human Error through Automation
Humans, while capable of incredible creativity, are also prone to errors and oversights.
AutoML reduces the reliance on manual intervention, thereby minimizing the chances of human error influencing model outcomes. This automated oversight ensures a higher degree of accuracy and reliability in model performance, making the development process both efficient and robust.
I apologize for the oversight in my previous response. Here’s the corrected version with the appropriate use of markdown for headings.
5. Improves Scalability of AI Solutions
In this section, we delve into how AutoML aids in managing the growing demands on AI systems, ensuring they remain efficient and manageable regardless of scale.
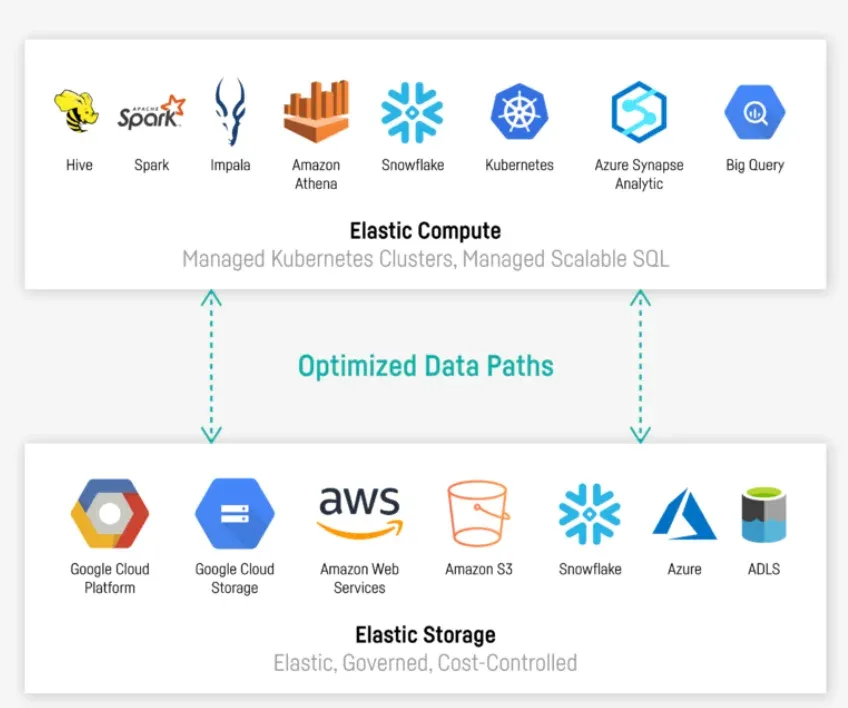
Efficient Resource Management and Allocation
AutoML introduces an era where AI can dynamically adjust to workload demands, optimizing the use of computational resources and ensuring projects remain scalable and manageable, no matter their size.
AutoML in Cloud Environments
The integration of AutoML within cloud environments signifies a leap towards limitless scalability, allowing systems to grow in capacity as data demands increase, without the hefty upfront investment in physical infrastructure.
Future-proofing AI Projects with AutoML
Embracing AutoML means equipping your AI projects with the ability to evolve.
As your data and operational complexities grow,
adapt seamlessly, keeping them relevant and efficient.
Scaling AI Solutions with Minimal Human Intervention
AutoML's greatest strength lies in its capacity to expand AI capabilities with minimal human oversight, allowing systems to intelligently adjust and scale based on real-time data and demands.
Suggested Reading: Building Scalable AI Solutions with AutoML
Adapting to Growing Data Sizes and Complexity
In an era where data is king, AutoML stands as the guardian of efficiency, ensuring AI systems can adapt and thrive as they encounter increasingly complex datasets and evolving operational demands.
Conclusion: The Future of AutoML in Data Science and AI
To recap, AutoML is setting the stage for a transformative shift in how AI and data science projects are executed.
Its impact on scalability, cost efficiency, and the support of continuous learning models speaks to a future where the development and deployment of AI are more inclusive, efficient, and adaptable.
Summary of Key Benefits
Embracing AutoML translates into significant advantages for those venturing into AI, enabling streamlined operations, reduced costs, and models that evolve with the landscape of data science.
The Evolving Landscape of AutoML Technologies
As we move forward, the advancements in AutoML technologies are poised to further redefine the boundaries of what's possible in AI and data science, making continuous learning and adaptation the new norm.
Challenges and Considerations for Adopting AutoML
Adopting AutoML comes with its set of challenges, from data privacy concerns to ensuring model transparency.
Understanding these factors is crucial for leveraging AutoML effectively and responsibly.
Parting Thoughts: The Strategic Edge of AutoML
Incorporating AutoML into your AI strategy can provide a significant edge, offering a path to more agile, cost-effective, and adaptive AI solutions that can stand the test of time and technological advancement.
Call to Action: Embracing AutoML for Future Projects
As we stand on the brink of this new era in AI and data science, the call to action is clear—embrace AutoML.
It’s time to leverage this powerful tool to ensure your projects are not just current but remain front-runners in the innovation race.
Frequently Asked Questions (FAQs)
How Does AutoML Benefit AI Model Development Time?
AutoML streamlines complex processes like model selection and hyperparameter tuning, significantly reducing the time required to develop an effective AI model.
Why is AutoML Useful for Non-Experts in AI and Data Science?
AutoML platforms have intuitive interfaces, abstracting the complexity for those with limited ML expertise, making it easier to build, deploy, and manage models.
How Can AutoML Enhance Model Performance?
AutoML automatically tests and compares different models on your data, selecting the one that delivers the best performance for your specific task, often outperforming manual approaches.
Does AutoML Aid in Model Interpretability?
Yes, many AutoML solutions incorporate explainability tools that help users understand how models make predictions, further demystifying the AI process.
How Does AutoML Advance Scalability in AI Projects?
AutoML simplifies and accelerates model deployment, making it easier to scale AI projects across different parts of a business and adapt to evolving business needs.