Introduction
Size isn't everything. That's especially true for language models. Bigger doesn't always mean better, and smaller doesn't always equate to less capable. These are the surprise twists in the burgeoning world of digital linguistics.
While sprawling, encyclopedia-like brains sound impressive, they're not always the smartest choice. Sure, they can dazzle with an ocean of words and ideas, but navigating that ocean can be a stormy affair. On the flip side, the trim, pocket-sized intellects of small language models cut through the waves with surprising agility.
This guide isn't about pitting David against Goliath in a coding coliseum. It's about understanding each model's place on the digital workbench. With large models, the wealth of knowledge comes with strings attached think hefty power consumption and the knack for absorbing quirks from their data diets. And the small fry? They may not spin out novels, but they'll dart through your day-to-day tasks with the finesse of a housecat chasing a laser dot.
Ease into this guide. It's a fresh take that demystifies these tools — laying out their strengths, exposing their weaknesses, and ultimately, steering clear of the techno-babble. By the end, the choice won't hinge on size. It'll be about fit, purpose, and the task at hand.
Understanding Language Models
Language models are like smart robots that understand and create human language. They can write stories, answer questions, or even chat like a friend. There are two main types: small and large models.
Small language models are like modest libraries. They know a bit, enough to help with basic tasks. They learn from fewer books (or data), making them quick learners but with a limited understanding. Think of them as younger students who know a lot about their favorite subjects but not much beyond that.
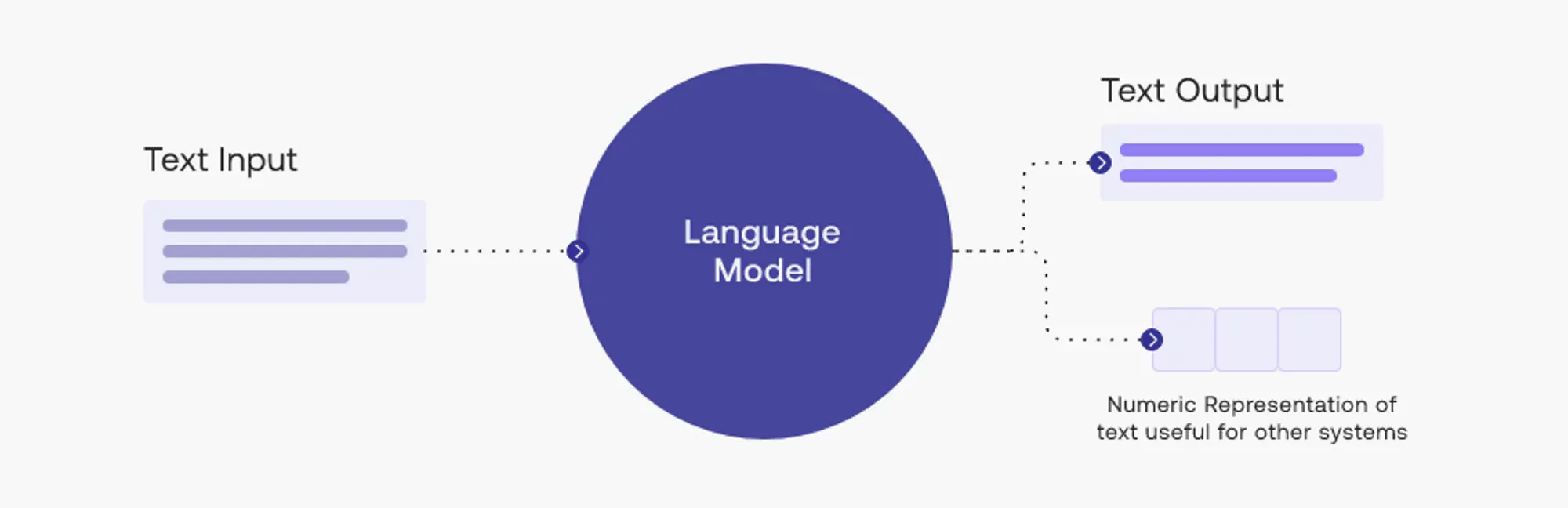
On the other hand, large language models are like vast libraries with millions of books. They've read so much that they can talk about almost anything. Because they've learned from more data, they're better at understanding complex ideas and making sense of difficult texts. Imagine them as scholars who have spent years studying various subjects.
The main difference between the two is how much they know and how well they understand language. Small models are quick and nimble, good for simple tasks. Large models are powerful and wise, great for tackling tough challenges.
Suggested Reading:Comparing Small Language Models to Large Scale Models
Strengths of Small Language Models
Small language models are like the little engines that could in the tech world. They may not know it all, but they're quick to set up and easy to use. Here's the lowdown on what makes them stand out:
- Quick to Train: They get up to speed fast, similar to prepping for a neighborhood 5K instead of a marathon. Less data to sift through means you're ready to go sooner.
- Low Resource Use: These guys are light on resources. No need for supercomputers; a basic setup does the trick, which is easier on the wallet and more eco-friendly too.
- Easy to Understand: With these models, it's simple to see what's happening under the hood. If you're teaching something new, you can follow along without getting lost.
- Simpler to Fix: When problems pop up, small language models are less of a headache to correct. It's like untangling your earbuds, not a 100-foot fishing net.
- Ideal for Specific Tasks: Some jobs don't need the big guns. Small models are perfect for straightforward tasks like writing a quick text or making a list.
Small language models might not be the wizard of all topics, but they have their own magic.
They train fast, are kind on the resources, super easy to get why they do what they do, simple to fix, and just right for the simpler stuff.
Limitations of Small Language Models
Small language models are super nifty, but they also have their hang-ups. Here are some areas where they sometimes stumble:
- Limited Knowledge Base: Compared to a large model, a small one has seen less of the world. It's like trying to have deep conversations with someone who only reads the daily news and hasn't dug into books on the subject.
- Incomplete Understanding: With complex topics, small models can struggle. It's like doing a jigsaw puzzle with missing pieces, they just can't give you the full picture.
- Struggling with Nuance: When you're dealing with tricky language stuff like poems or jokes, small models may not always get it. It’s like explaining a joke; if you have to explain it, it’s not that funny. Small models often need the joke explained to them.
- Repetitive: Sometimes, they can sound like a broken record. In a conversation, or when asking them for creative ideas, they might keep hitting the same notes.
While small models are neat, they also have their challenges. They might not know as much, struggle with complex stuff, not pick up on nuanced language, and can be a bit repetitive. But hey, nobody’s perfect!
Suggested Reading:Comparing Small Language Models to Large Scale Models
Strengths of Large Scale Models
Big language models have some exciting advantages. Despite their huge appetite for resources and time, these models are like supercomputers of language understanding. Here's a snapshot of what they bring to the table:
- Rich Understanding: Since large models feast on a lot of data, they have a deep understanding of language. It's like having a conversation with someone who has read every book in the world, understands every line and reference. This makes them super-smart and capable.
- Versatility: Big models are like Swiss army knives of language tasks. They can write essays, translate languages, even create poetry or jokes. They're quite the multi-taskers, capable of doing many things well.
- High Accuracy: Because they've learned from more data, large models hit the bulls-eye more often. They can read complex text, understand it, and give accurate responses. They're like seasoned detectives who spot important clues easily.
- Contextual Relevance: Large models are great at understanding context. They manage to keep the thread of a conversation better, like a good friend who remembers what you discussed last time.
- Large Knowledge Base: Large models have learned from probably the most comprehensive library imaginable. They can chat about almost any topic under the sun, often surprising us with their range of knowledge.
So, despite being resource-hungry, large models shine where it matters. They have rich knowledge, multi-task with ease, hit the mark with accuracy, understand context really well, and boast a vast reservoir of knowledge.
Limitations of Large Scale Models
Big language models may seem like the superheros of the language world, but they come with their own kryptonite. Here's a look at the drawbacks of these models:
- Resource Intensive: Large models are like high-performance sports cars. They're stunning to drive, but they guzzle gas and demand frequent expensive tune-ups. In other words, they need powerful computers and eat up a lot of electrical power, making them pricey to run.
- Time Consuming: Training large models is like climbing Mount Everest. Sure, the view from the top is spectacular, but it takes a lot of time, preparation, and hard work to get there.
- Tricky to Tweak: Fine-tuning big models is a bit like navigating a cruise ship. Changing direction takes time and a lot of effort. Meaning, if you want to adjust them slightly for specific tasks, it won't be quick or easy.
- Risk of Biases: Like learning from a book that has some incorrect info, large models can pick up and reinforce biases present in their training data. They're kind of like sponges, soaking up everything, even the uncool stuff.
So even though large language models are powerhouses of text-generation, they're not without quirks. They need lots of resources, take time to train, demand patience when you want to tweak them, and can sometimes repeat things they shouldn't.
Conclusion
Alright, so that's the scoop on large and small language models. The big models are brainiacs, sure. They have a vast knowledge, can multitask, are pretty precise, understand context, and cover almost every topic you can think of.
But they're also resource heavy, take time, demand a lot when you want to finetune them, and sometimes share things they shouldn't.
Then there are the small models. They're quick, light on resources, easy to understand and fix, and ideal for straightforward tasks. But they might know less, get puzzled by complex stuff, not always grasp nuanced language, and can sound like they're stuck on repeat.
It's a mix of pros and cons really. Some scenarios need the heavyweight champ, some just require the light and agile contender. Choose your fighter wisely.
Frequently Asked Questions (FAQs)
Can language models learn over time?
Yes, they can improve with updates and training, but they don't learn autonomously after deployment.
Are small language models better for privacy?
They can be, as they process less data and often work locally, reducing data exposure.
How do cost differences impact the choice between models?
Smaller models are cheaper to run and maintain, while larger ones usually require more investment.
Do all language models require an internet connection?
Not all. Some small models can run offline, while larger models often need online resources.