Generative AI is on the cusp of transforming countless industries, sparking intrigue and inspiration. The creative potential of AI algorithms has been capturing imaginations worldwide. But what is generative AI, exactly? And how can it unleash endless possibilities in unprecedented ways?
As breakthroughs in technology reshape the way we live and work, understanding the ins and outs of generative AI becomes vital. These AI systems are already churning out unique artworks, medical breakthroughs, and video game assets. But like any groundbreaking technology, generative AI brings its challenges and limitations to the forefront.
To truly grasp the power of generative AI, a comprehensive exploration of its applications, use cases, and complexities is essential. Whether you're an industry insider or simply curious about the future of AI, this guide aims to provide you with an accessible understanding of the subject.
By the end of this journey, you'll realize that generative AI's impact goes beyond just creating new content. It alters the ways humans and machines collaborate, unlocking opportunities, and solving problems on a grander scale. So, buckle up and get ready for a deep dive into the world of generative AI.
Overview of Generative AI
Generative AI is a buzzword these days, but what is generative AI? Think of it as a creative brain. It's a type of artificial intelligence—specifically, machine learning—that creates new data from the data it's been trained on.
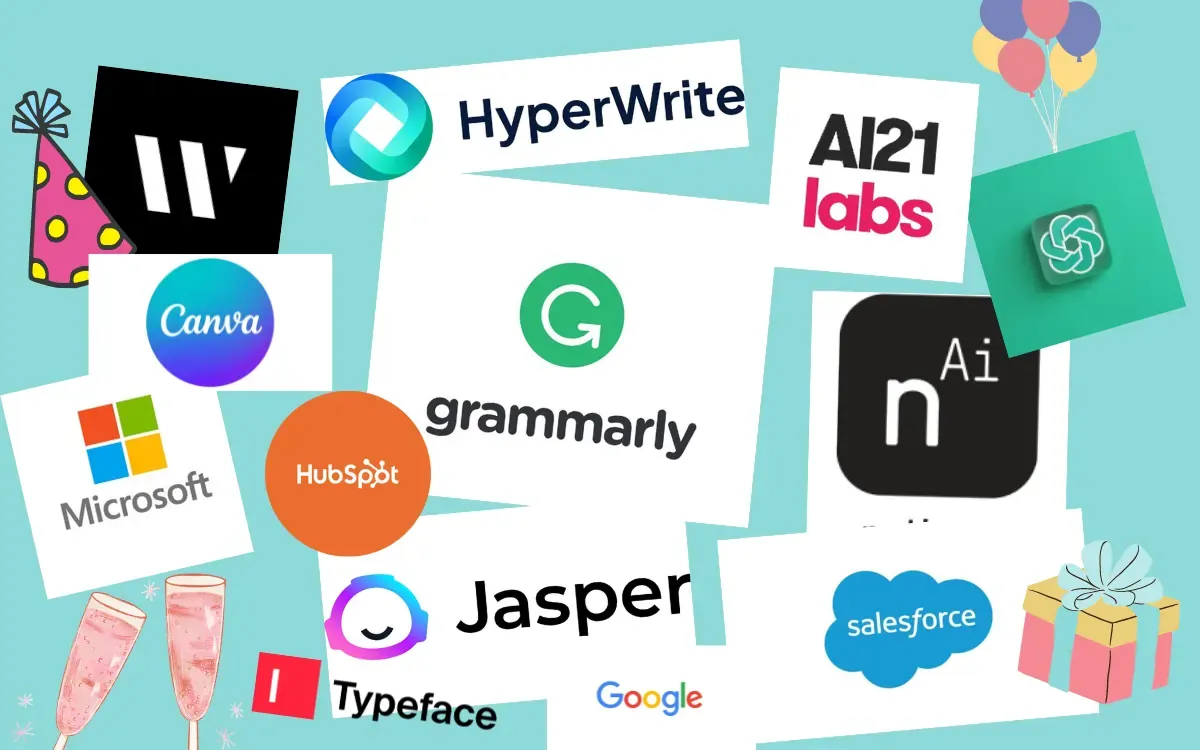
To understand how does generative AI work, consider a potter shaping clay. Just as a potter forms a unique vessel from a lump of clay, generative AI uses a stack of algorithms to mold unique outputs from huge piles of data. It learns patterns, nuances, and structures from existing data.
Then, it generates new, unseen data that mirrors the original. This is known as a "generative AI model." It can create music, write poetry, or even help design new products.
Numerous generative AI companies are emerging in this space, offering unique and innovative generative AI tools. They are disrupting the AI-industry with sophisticated technologies. Whether you're at a tech-startup or an industry giant—everyone is jumping on the generative AI bandwagon.
Generative AI has applications in several fields. In customer service, it can autogenerate responses to customer queries. Artists are using it to create unique artworks. Generative AI in healthcare is another big application field. AI models are being developed to simulate the spread of diseases or help in drug discovery. These are just a few of the compelling generative AI use cases out there.
Generative AI is an AI method that learns from data and creates new, similar content. Its potential applications are vast and exciting. But, where does it stumble? We'll discover this in the upcoming sections.
Generative AI Applications and Use Cases
Generative AI is spreading its wings in multiple domains. Let's look at some noteworthy generative AI applications and use cases in different sectors.
Art and design: One of the popular applications of generative AI is in art and design. Creatives use generative AI tools to produce innovative, unique artwork, and designs that can surprise even the artist themselves!
It works by learning from existing samples and generating fresh ideas based on underlying patterns.
Text generation: What is generative AI's role in text generation? From writing news articles to product descriptions, generative AI tools can craft coherent, contextually relevant text.
These AI systems analyze vast collections of writing samples, understand the grammatical structures, and create new text that feels almost human-authored.
Music composition: Generative AI has the potential to revolutionize the music industry. By studying patterns, rhythm, and musical notes, generative AI can create original tunes, opening up a whole new world of possibilities for composers and performers alike.
Generative AI in healthcare: The healthcare industry is witnessing the transformative power of generative AI. AI models help in drug discovery by creating virtual molecules that can potentially fight diseases.
It's also used for medical imaging purposes, such as enhancing low-resolution images or reconstructing 3D models from 2D scans. These applications help accelerate research and development, opening new avenues for medical breakthroughs.
Video game development: Gaming is another industry benefiting from generative AI applications. AI tools can create in-game assets, such as characters, landscapes, or even generate dialogues for non-player characters, enhancing the gaming experience beyond imagination.
Cybersecurity: Generative AI is also stepping into the world of cybersecurity. It aids in simulating cyber-attacks, helping organizations identify vulnerabilities in their systems. It's becoming an essential component of cyber defense strategies.
As generative AI companies race to develop innovative solutions, businesses across industries will continue to explore new use cases. However, all that glitters is not gold. Generative AI tools are complex, often resource-intensive, and—as we'll discuss in the next section—come with some inherent limitations.
The key is to balance the opportunities offered by generative AI with its potential challenges and forge a path for a better technological future.
Generative AI is no magic wand, but it's already transforming the way we engage with creativity, science, and technology. And the best part? We're only just getting started. As we dive deeper into understanding how does generative AI work, we open doors to infinite possibilities for human-AI collaboration.
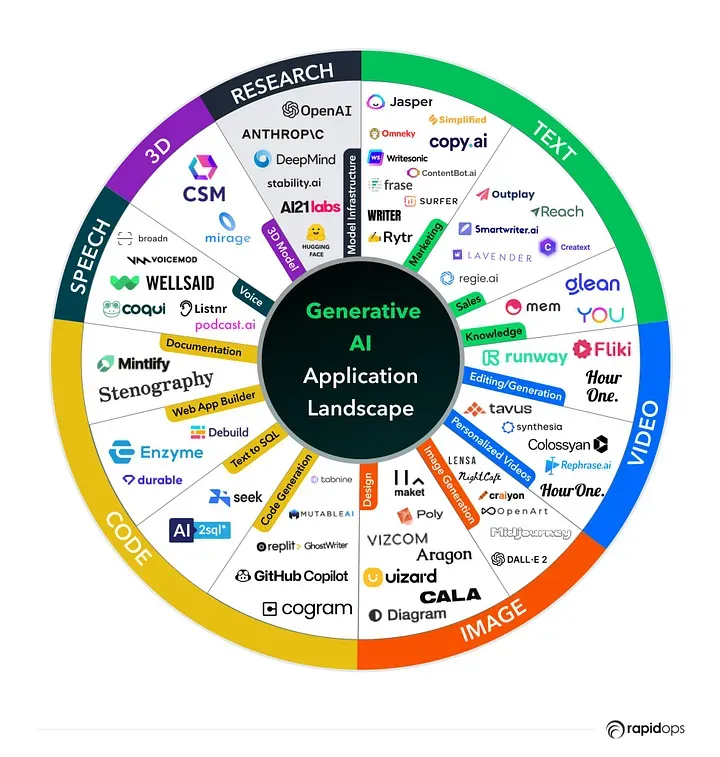
Challenges and Limitations of Generative AI
Generative AI holds enormous potential, as we've explored its diverse applications. However, it's not without its hurdles. In this section, we'll shed light on some challenges and limitations that generative AI faces. Understanding these aspects is as crucial as appreciating the potential of this technology.
By knowing these points, we can better harness the power of generative AI and mitigate the risks that come with it.
Challenges
Before diving into the challenges of generative AI, let's briefly introduce what these challenges entail. Essentially, these are obstacles that generative AI companies and developers face while working on creating or implementing these AI systems. They often impact the efficiency of the generative AI model or involve ethical concerns surrounding AI usage.
- Data quality and quantity: Generative AI tools require large datasets to learn patterns and generate accurate outputs. Companies often struggle to acquire high-quality, diverse, and unbiased data, which impedes the performance of AI models.
- Computational resources: Training generative AI models demands significant computational power, which can be expensive and resource-intensive. This barrier poses a challenge for small businesses or individuals looking to leverage generative AI applications.
- Long development cycles: Developing, training, and optimizing generative AI models can take time. The complexity involved in the process demands extensive research and development cycles, which challenge the pace of innovation.
- Lack of interpretability: Generative AI models can be hard to understand and interpret. The "black box" nature of AI systems makes it difficult to identify errors, explain outputs, or validate the decision-making process. This lack of transparency can hamper trust and adoption.
- Ethical considerations: The rise of generative AI has sparked ethical questions, especially around content ownership and intellectual property. Plus, concerns about the potential misuse of AI-generated content, such as deepfakes or fake news, have made it necessary to establish ethical guidelines and regulations for its use.
- Talent scarcity: There's a significant talent gap due to the high demand for machine learning experts. This shortage challenges the growth of generative AI companies and the development of innovative applications.
Limitations
Now that we've covered the challenges, let's turn our attention to the limitations of generative AI. Unlike challenges, limitations are not related to the external factors of AI development but stem from the nature of AI technology itself. Here, we'll discuss some inherent constraints within generative AI applications.
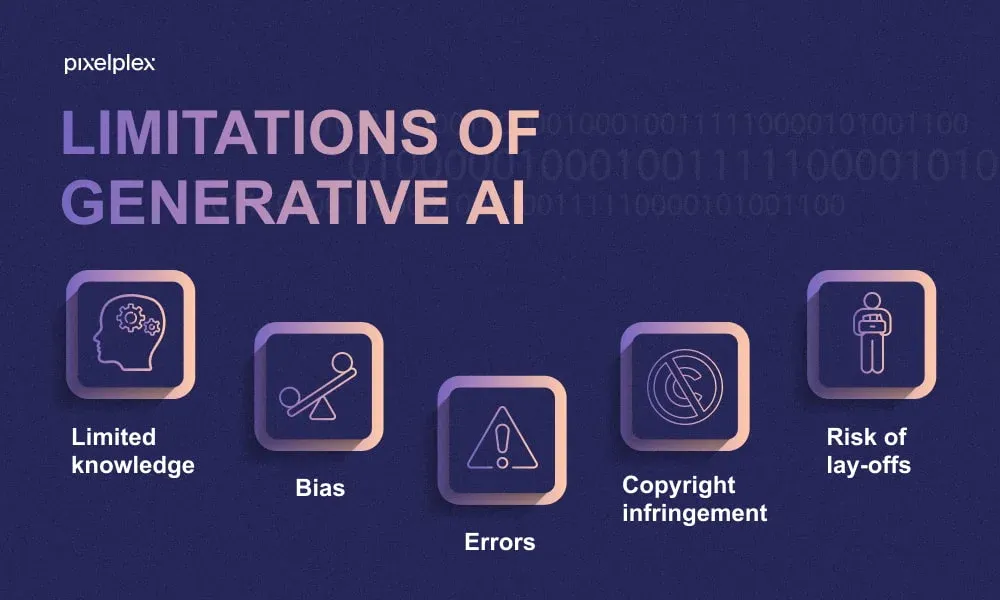
- Limited creativity: While generative AI applications can produce impressive results, their capacity for creativity remains restricted. AI models will always depend on the input data they receive, so the outputs are confined to the patterns they've learned. Human intuition, spontaneity, and innovation still surpass AI-generated creativity.
- Overfitting: Generative AI models can become overly specific to the training data, causing overfitting. It reduces the model's flexibility to generate fresh outputs, as the AI closely adheres to the training data patterns.
- Unintentional bias: AI models may pick up on and replicate existing biases in the training data. This factor can lead to outputs that perpetuate stereotypes and prejudices, unintentionally causing harm or reinforcing existing inequalities.
- Lack of common sense: Generative AI tools may produce grammatically correct and contextually relevant content, but they lack an understanding of the real world. Consequently, they might generate outputs that make no practical sense or contradict established facts.
- Quality control: Ensuring the quality of AI-generated content remains a significant limitation. It can be challenging to measure the reliability, relevance, and accuracy of the outputs produced. Users often need to review AI-generated content and rectify any flaws manually.
Generative AI carries enormous potential for various applications across industries. However, it's crucial to understand and navigate the challenges and limitations that come with it.
By staying aware of these factors, we can harness the power of generative AI responsibly and optimally, pushing the boundaries of human-machine collaboration and creating a future that benefits all.
Conclusion
In this guide, we explored the fascinating world of generative AI, its applications, and potential challenges and limitations. As AI continues to advance, we're bound to witness even more innovative solutions across various sectors.
However, it's important not to overlook the hurdles we need to overcome. A responsible approach to AI development should carefully consider ethical aspects and be mindful of the technological constraints at play.
By doing so, we can strike a balance between leveraging generative AI's power and mitigating its risks, paving the way for a future that offers endless possibilities and fosters genuinely meaningful human-AI collaboration.
Frequently Asked Questions (FAQs)
Are there any successful commercial products based on generative AI?
Indeed, several successful commercial products leverage generative AI technology.
For instance, chatbots in customer service, music generators in the entertainment industry, and content creation software in digital marketing all benefit from generative AI.
How secure is generative AI?
Generative AI's security largely depends on its implementation. Appropriate data protection measures and ethical guidelines should be in place to prevent misuse and ensure security.
Can I learn generative AI without a programming background?
While a programming background certainly helps, many introductory courses can teach the basics of AI and machine learning without requiring extensive coding knowledge.
What are the future trends in generative AI?
Future trends in generative AI could include more personalized content creation (such as AI-driven music or art compositions tailored to individual tastes), increased use in healthcare research, and progressing towards more sophisticated and creative AI models.